A high school is composed of 400 students who are either freshman, sophomores, juniors, or seniors. Using this list, you stratify on two characteristics: Example of stratified random sampling.
Probability Sampling Methods Explained with Python by 👩🏻
First, she splits the population of interest into two strata based on gender so that we have 4,000 male students and 6,000 female students.
Create the dummy dataset from a python dictionary using pandas dataframe.
Following is a classic stratified random sampling example: For example, if the larger population contains 40% history majors and 60% english majors, the final sample should reflect these percentages. When stratifying, researchers tend to use proportionate sampling where they maintain the correct proportions to represent the population as a whole. At the end of section 6.3, we discuss stratified sampling for proportions.
Suppose a research team wants to determine the gpa of college students across the u.s.
Let’s say, 100 (nh) students of a school having 1000 (n) students were asked questions about their favorite subject. The population mean (μ) is estimated with: Hope now it’s clear for all of you. It’s a fact that the students of the 8th grade will have different subject.
Suppose a research team wants to cause the gpa of college students across the united states.
In stratified sampling, researchers divide subjects into subgroups called strata based on characteristics that they share (e.g., race, gender, educational attainment. Example of stratified random sampling a research team performed a study on the gpas of trade school students across the state of california. A stratified random sample is a population sample that requires the population to be divided into smaller groups, called 'strata'. For example, people’s income or education level is a variation that can provide an appropriate backdrop for strata.
In this example, we have a dummy dataset of 10 students and we will sample out 6 students based on their grades, using both disproportionate and proportionate stratified sampling.
In stratified random sampling, any feature that explains differences in the characteristics of interest can be the basis of forming strata. This means that every element in the population must be assigned to only one stratum, and there shouldn’t be any overlap of. A stratified sample of the same size can provide more accuracy than a simple random sample. This tutorial explains how to perform stratified random sampling in r.
Let’s move on to our next approach i.e.
In contrast, stratified random sampling divides the. In section 6.3, we use an example to illustrate that a stratified sample may not be better than simple random sample if the variable one stratifies on is not related to the response. You compile a list of every graduate’s name, gender identity, and the degree that they obtained. S stratified sample often necessitates a smaller sample size because it offers better precision, which saves money.
The example of stratified random sampling.
In stratified sampling, the population to be sampled is dividedinto groups (strata), and then a simple random sample from eachstrata is selected. They took a random selection of 2,000 trade school students out of the 10.5 million students in the state. Example of proportionate stratified sampling as part of a research to know how many students want to pursue a career in the sciences. This would be our strategy in order to conduct a stratified sampling.
Gender identity, with three strata (male, female, and other), and degree, with.
Therefore, it decides to sample the population by using 4,000 students indiscriminately. For example, a state could be separated intocounties, a school could be separated into grades. It also helps them obtain precise estimates of each group’s characteristics. The population is first divided into homogeneous subpopulations, or stratas, that are mutually exclusive and collectively exhaustive.
There are several advantages to stratified sampling placed above a white simple random sampling (brink, m et al.,2019).
With only one stratum, stratified random sampling reduces to simple random sampling. In statistical analysis, the population is the total set of observations or data. Stratified sampling is a method of obtaining a representative sample from a population that researchers have divided into relatively similar subpopulations (strata). The research team has difficulty collecting data from all 21 million.
This is an example of stratified sampling.
()∑ = = + + + = l i n n nl l n ni i n 1 1 1 2 2 1 1 μˆ μˆ μˆ l μˆ μˆ where n i is the total number of sample units in strata i, l is the number of strata, and n is the total How do you use stratified sampling? In stratified sampling, a sample is drawn from each strata (using a random sampling method like simple random sampling or systematic sampling). Moreover, the research team has difficulty in collecting data from all 21 million college students.
Suppose we’d like to take a stratified sample of 40 students such that 10 students from each grade are included in the sample.
Cluster sampling and stratified sampling share the following similarities:
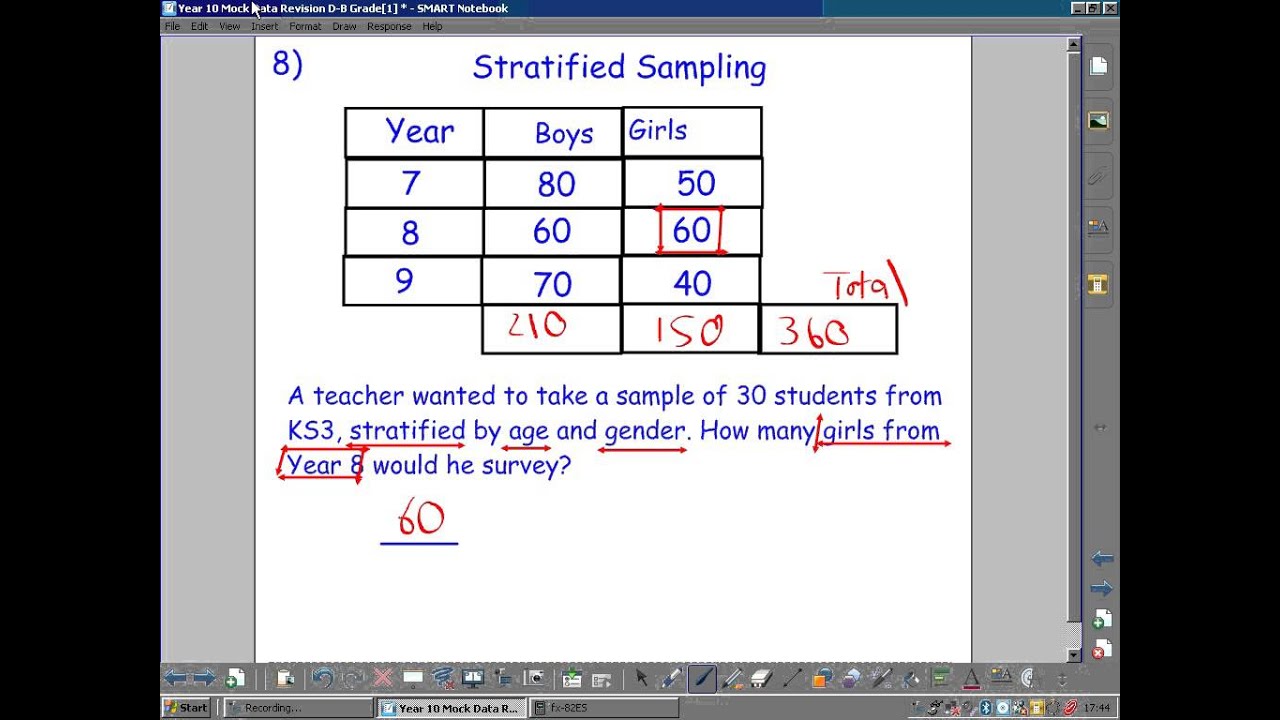

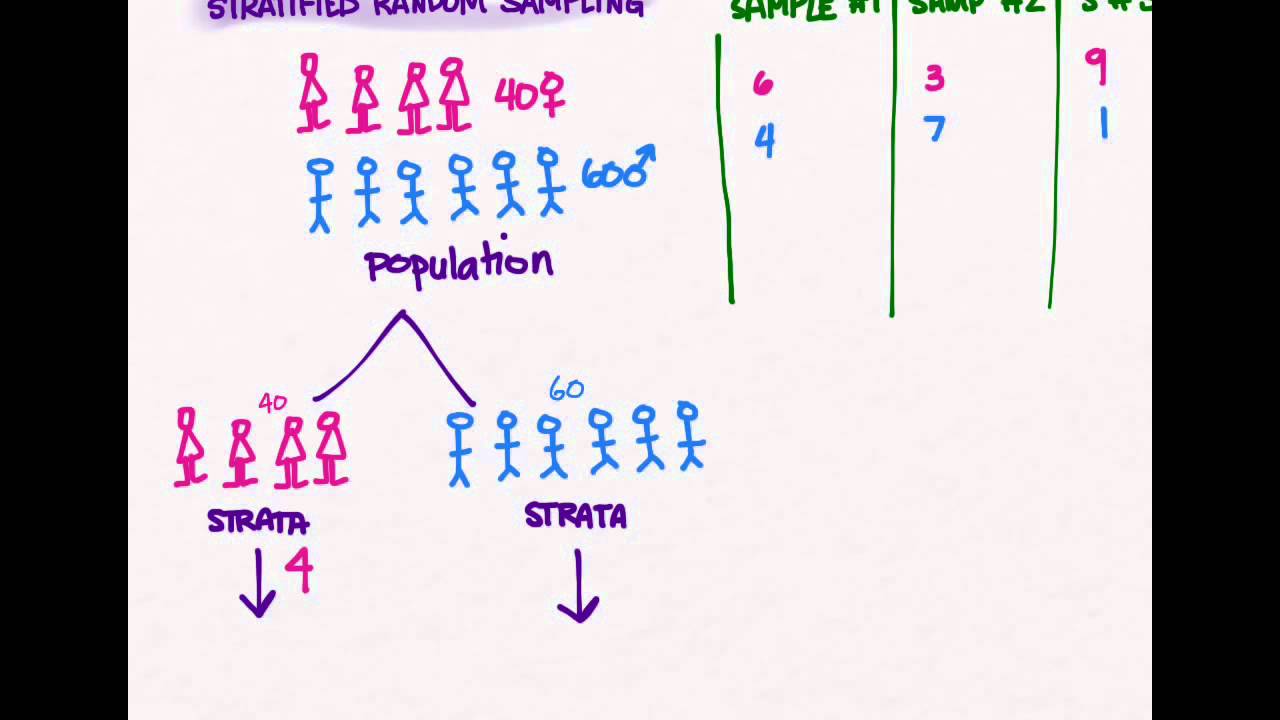



