The term multicollinearity was first used by ragnar frisch. This correlation is a problem because independent variables should be independent.if the degree of correlation between variables is high enough, it can cause problems when you fit the model and interpret the results. In our loan example, we saw that x1 is the sum of x2 and x3.
Tut9 multicollinearity
They can become very sensitive to small changes in the model.
When several independent variables are highly but not perfectly correlated among themselves, the regression result is unreliable, this phenomenon is known as multicollinearity, and as a consequence, we are not able to disprove the null hypothesis, wherein we should actually reject the same.
Multicollinearity refers to a situation at some stage in which two or greater explanatory variables in the course of a multiple correlation model are pretty linearly related. Multicollinearity occurs when an explanatory variable is strongly related to a linear combination of the other independent variables. Is multicollinearity a problem in logistic regression? Linear regression analysis assumes that there is no perfect exact relationship among exploratory variables.
Multicollinearity is a common problem when estimating linear or generalized linear models, including logistic regression and cox regression.it occurs when there are high correlations among predictor variables, leading to unreliable and unstable estimates of regression coefficients.
The analysis exhibits the signs of multicollinearity — such as, estimates of the coefficients vary excessively from model to model. Perfect (or exact) multicollinearity if two or more independent variables have an exact linear relationship between them then we have perfect multicollinearity. This occurs when at least two predictor variables have an exact linear relationship between them. It is predicted by taking a variable and regressing it against every other variable.
The most extreme case of multicollinearity is known as perfect multicollinearity.
The variables are independent and are found to be correlated in some regard. Multicollinearity occurs when independent variables in a regression model are correlated. ” vif determines the strength of the correlation between the independent variables. In regression analysis, when this assumption is violated, the problem of multicollinearity occurs.
Multicollinearity is a term used in data analytics that describes the occurrence of two exploratory variables in a linear regression model that is found to be correlated through adequate analysis and a predetermined degree of accuracy.
The column rank of a matrix is the number of linearly independent columns it has. So, in this case we cannot exactly trust the coefficient value (m1).we don’t know the exact affect x1 has on the dependent variable. Read more in the equation predict the perfect linear relationship. What are the problems that arise out of multicollinearity?
The end objective) that is measured in mathematical or statistical or financial modeling.
Notice that the values for predictor variable x2 are simply the values of x1 multiplied by 2. Structural multicollinearity is a mathematical artifact caused by creating new predictors from other predictors — such as creating the predictor x 2 from the predictor x. For example, suppose we have the following dataset: This increase means that the parameter estimates are less reliable.
Multicollinearity generates high variance of the estimated coefficients and hence, the coefficient estimates corresponding to those interrelated explanatory variables will not be accurate in giving us the actual picture.
In this situation, the coefficient estimates of the multiple regression may change erratically in response to small changes in the model or the data. Multicollinearity occurs when there is a high correlation between the independent variables in the regression analysis which impacts the overall interpretation of the results. For illustration, we take a look at a new example, bodyfat.this data set includes measurements of 252 men. Because of this relationship, we cannot expect the values of x2 or x3 to be constant when there is a change in x1.
In statistics, multicollinearity (also collinearity) is a phenomenon in which one predictor variable in a multiple regression model can be linearly predicted from the others with a substantial degree of accuracy.
The goal of the study was to develop a model, based on physical measurements, to predict percent body fat. The meaning of multicollinearity is the existence of such a high degree of correlation between supposedly independent variables being used to estimate a dependent variable that the contribution of each independent variable to variation in. Variance inflation factor (vif) measures the degree of multicollinearity or collinearity in the regression model. Multicollinearity does not violate the assumptions of the model, but it does increase the variance of the regression coefficients.
Multicollinearity can be detected via various methods.
It describes a perfect or exact relationship between the regression exploratory variables.

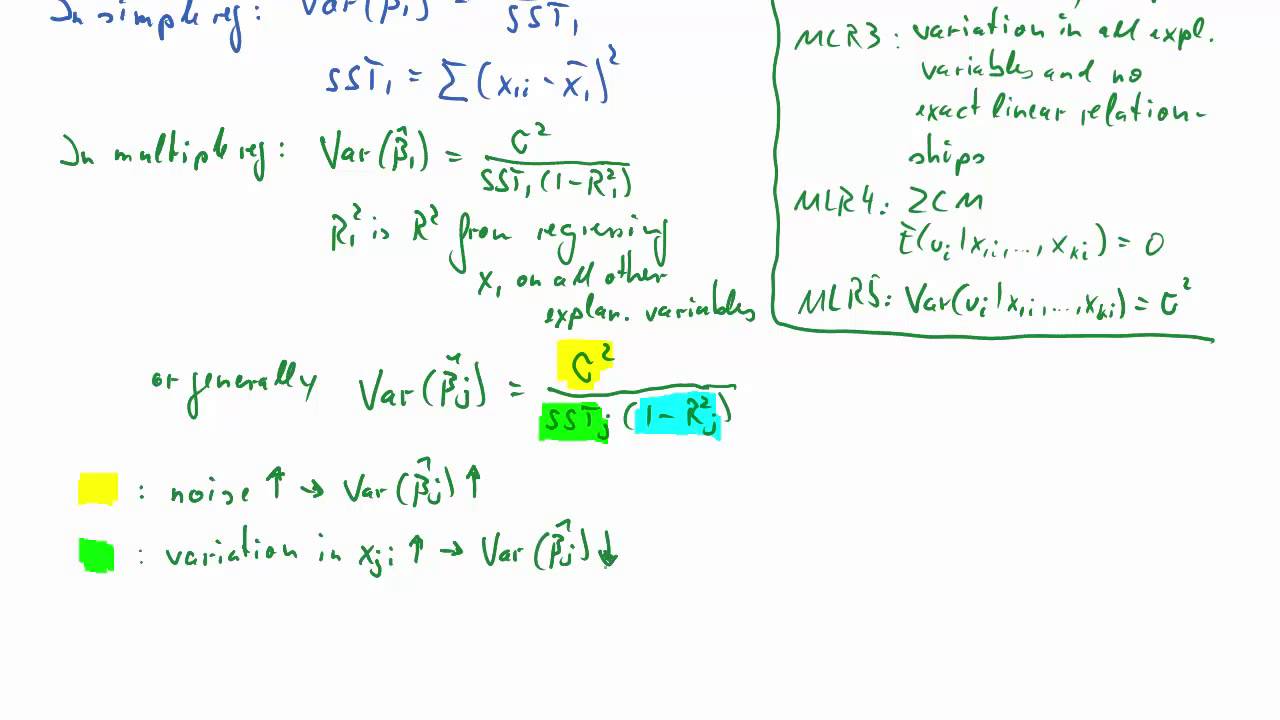




