• multicollinearity inflates the variances of the parameter estimates and hence this may lead to lack of statistical significance of individual predictor variables even though the overall model may be significant. Continuing the example stated above, the presence of multicollinearity is examined in the model stating that customer loyalty is affected by customer satisfaction, product quality, service quality, and brand awareness. If the degree of correlation is high enough between variables, it can cause problems when fitting and interpreting the regression model.
SPSS v.23 Lesson 56 اختبار التعددية الخطية
• the presence of multicollinearity can cause serious problems with the estimation of β and the interpretation.
Turn on the spss program and select the variable view, furthermore, in the name write competency, motivation, performance.
I am trying to conduct an ordinal logistic regression, but i first want to test if i fulfill the assumption of no multicollinearity. Tolerance is a measure of collinearity reported by most statistical programs such as spss; Why do we test for autocorrelation? An enhancement request has been filed to request that collinearity diagnostics be added as options to other procedures, including logistic regression, nomreg, and plum.
Multicollinearity refers to when your predictor variables are highly correlated with each other.
Multicollinearity test via pearson’s correlation coefficient The next step, click the data view and enter research data in accordance with the variable. Multicollinearity means independent variables are highly correlated to each other. Learn how to detect and handle with multicollinearity in spss.
All the data pertain to the most recent 12 months for which data were available for each company.
Technote #1476169, which is titled recoding a categorical spss variable into indicator (dummy) variables, discusses how to do this. Multicollinearity generally occurs when there are high correlations between two or more predictor variables. This is an issue, as your regression model will not be able to accurately associate variance in your outcome variable with the correct predictor variable, leading to muddled results and incorrect inferences. However, this is required that dv should be continuous data.
How do you test for multicollinearity in spss linear regression?
One way to detect multicollinearity is by using a metric known as the variance inflation factor (vif), which measures the correlation and strength of correlation between the predictor variables in a regression model. Review the correlation matrix for predictor variables that correlate highly. The analysis was done using spss software. How to test multicollinearity using spss?
In other words, one predictor variable can be used to predict the other.
An easy way to detect multicollinearity is to calculate correlation coefficients for all pairs of predictor variables. A small tolerance value indicates that the. Computing the variance inflation factor (henceforth vif) and the tolerance statistic. It was suggested to use to test collinearity by a stats faculty member, and the idea is as below:
Bring up your data in spss and select.
Computing the variance inflation factor (henceforth vif) and the tolerance statistic. Turn on the spss program and select the variable view, furthermore, in the name write competency, motivation, performance. If the degree of correlation is high enough between variables, it can cause problems when fitting and. Multicollinearity in regression analysis occurs when two or more predictor variables are highly correlated to each other, such that they do not provide unique or independent information in the regression model.
There are three diagnostics that we can run on spss to identify multicollinearity:
The next step, click the data view and enter research data in accordance with the variable competency, motivation, performance. All of my 8 independent variables are ordinal with up to 5 levels. We can request that spss run all these diagnostics simultaneously. You can assess multicollinearity by examining tolerance and the variance inflation factor (vif) are two collinearity diagnostic factors that can help you identify multicollinearity.
How to test for multicollinearity in spss statology?
Using spss, multicollinearity test can be checked in linear regression model. How to test for multicollinearity in spss. Step by step to test multicollinearity using spss. Step by step to test multicollinearity using spss 1.
In this video i show how to conduct a multicollinearity test (with vifs) in spss.
However, this is required that dv should be continuous data. How to test for multicollinearity in spss logistic regression identifying multicollinearity in multiple regression statistics help for dissertation students & researchers how to identify multicollinearity you can assess multicollinearity by examining tolerance and the variance inflation factor (vif) are two collinearity diagnostic factors that can help you identify Multicollinearity in regression analysis occurs when two or more explanatory variables are highly correlated to each other, such that they do not provide unique or independent information in the regression model. Am i correct in thinking these need to be converted into dummy variables, modelled, and then the vif calculated?
There are three diagnostics that we can run on spss to identify multicollinearity:
The accompanying data set presents simulated financial data of some companies drawn from four different industry sectors. Using spss, multicollinearity test can be checked in linear regression model.


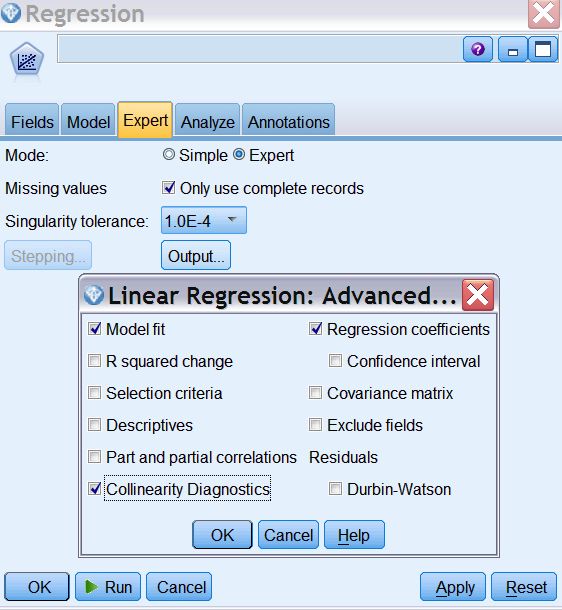

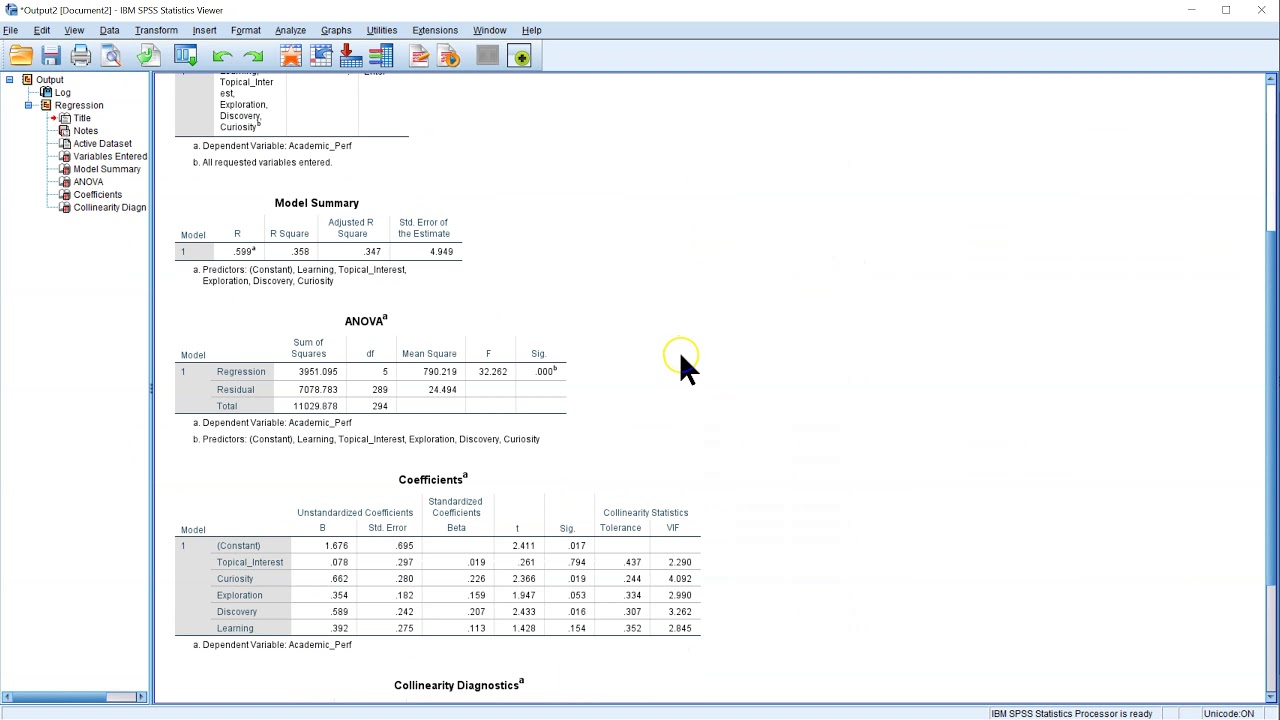

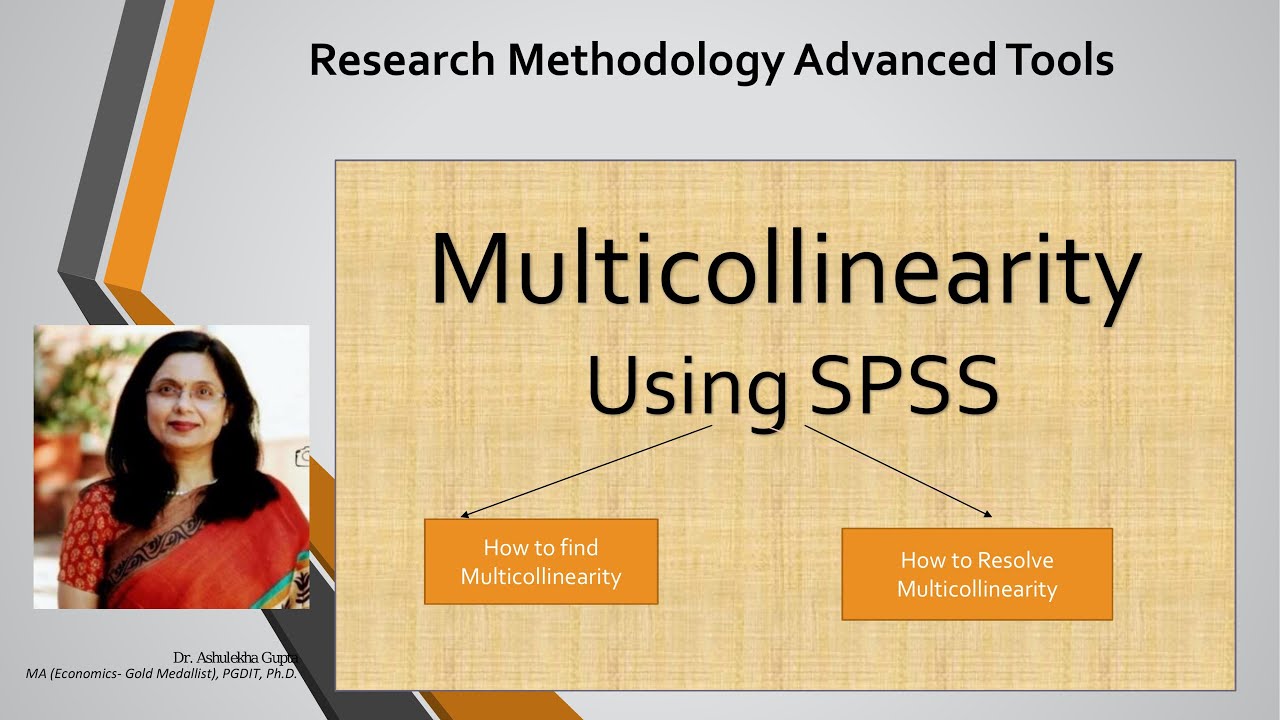