Multicollinearity occurs when independent variables in a regression model are correlated. A guide to multicollinearity & vif in regression. And below this table appears another table with the title collinearity.
Multiple Linear Regression in SPSS with Assumption Testing
How do you test for multicollinearity in spss linear regression?
Multicollinearity is a common problem when estimating linear or generalized linear models, including logistic regression and cox regression.
If the degree of correlation is high enough between variables, it can cause problems when fitting and. In a few cases, the variables can be highly. There are three diagnostics that we can run on spss to identify multicollinearity: However, this is required that dv should be continuous data.
There are three diagnostics that we can run on spss to identify multicollinearity:
This means that essentially \({{x}_{1}}\) is a linear function of \({{x}_{2}}\). It occurs when there are high correlations among predictor variables, leading to unreliable and unstable estimates of. In this section, we will explore some spss commands that help to detect multicollinearity. This paper discusses on the three primary techniques for detecting.
First, in the coefficients table on the far right a collinearity statistics area appears with the two columns tolerance and vif.
Multicollinearity refers to when your predictor variables are highly correlated with each other. I'm using the binary logistic regression procedure in spss, requesting the backwards lr method of predictor entry. Y= b0 + b1x1 + b2x2. Before analyzing any set of variables in a linear model, including logistic regression, begin by check for multicollinearity by using linear regression to check the model.
Does this procedure have any mechanism for assessing multicollinearity among the predictors and removing collinear predictors before the backward lr selection process begins?
The next step, click the data view and enter research data in accordance with the variable competency, motivation, performance. First, in the coefficients table on the far right a collinearity statistics area appears with the two columns tolerance and vif. This correlation is a problem because independent variables should be independent. We obtain the following results:
We can request that spss run all these diagnostics simultaneously.
Review the correlation matrix for predictor variables that correlate highly. By jim frost 185 comments. Computing the variance inflation factor (henceforth vif) and the tolerance statistic. Multicollinearity occurs when the multiple linear regression analysis includes several variables that are significantly correlated not only with the dependent variable but also to each other.
Jan 18, 2020 · if the option collinearity diagnostics is selected in the context of multiple regression, two additional pieces of information are obtained in the spss output.
If the option collinearity diagnostics is selected in the context of multiple regression, two additional pieces of information are obtained in the spss output. Computing the variance inflation factor (henceforth vif) and the tolerance statistic. Multicollinearity in regression analysis occurs when two or more predictor variables are highly correlated to each other, such that they do not provide unique or independent information in the regression model. In statistics, multicollinearity (also collinearity) is a phenomenon in which one predictor variable in a multiple regression model can be linearly predicted.
Tolerance is a measure of collinearity reported by most statistical programs such as spss;
If the degree of correlation is high enough between variables, it can cause problems when fitting and. Using spss, multicollinearity test can be checked in linear regression model. This usually caused by the researcher or you while creating new predictor variables. You can assess multicollinearity by examining tolerance and the variance inflation factor (vif) are two collinearity diagnostic factors that can help you identify multicollinearity.
How to test for multicollinearity in spss.
Multicollinearity in regression analysis occurs when two or more predictor variables are highly correlated to each other, such that they do not provide unique or independent information in the regression model. Turn on the spss program and select the variable view, furthermore, in the name write competency, motivation, performance. Review the correlation matrix for predictor variables that correlate highly. This is an issue, as your regression model will not be able to accurately associate variance in your outcome variable with the correct predictor variable, leading to.
Multicollinearity makes some of the significant variables under study to be statistically insignificant.
This is generally caused due to the experiments designed poorly, methods of collection of data which cannot be manipulated, or purely observational data. This indicates that most likely we’ll find multicollinearity problems. Now we run a multiple regression analysis using spss. If the degree of correlation between variables is high enough, it can cause.




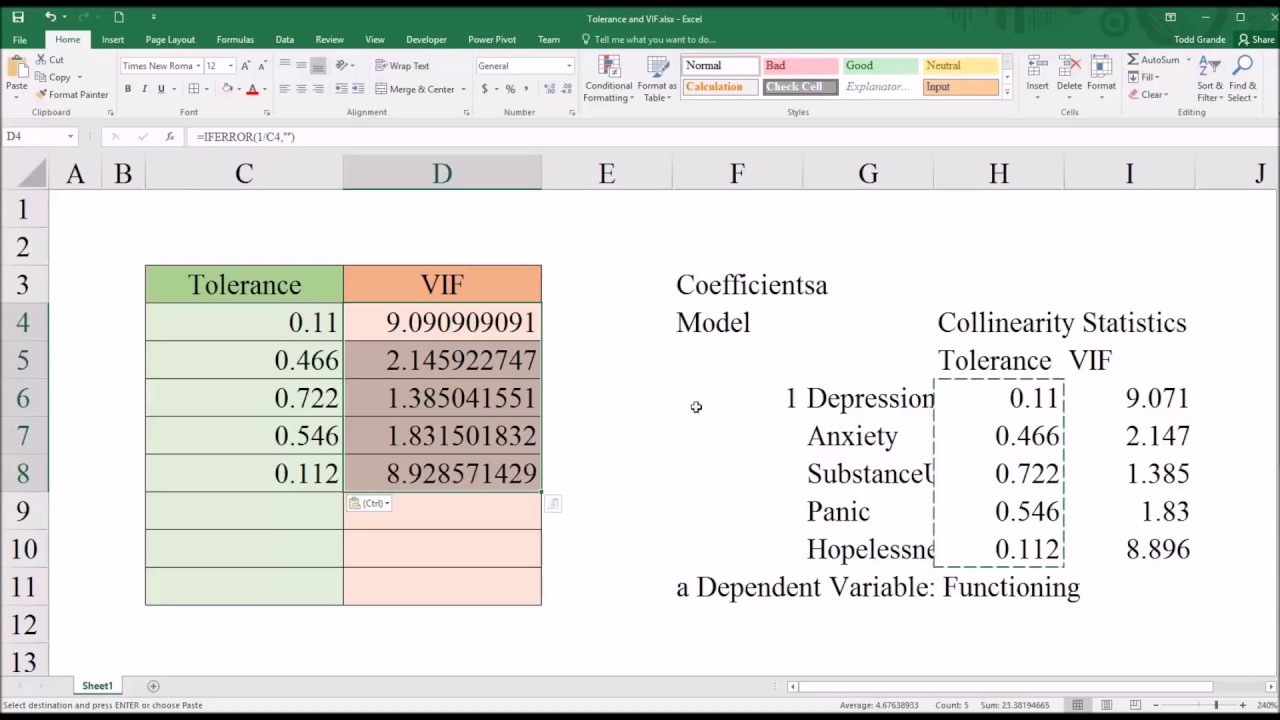
