This is an issue, as your regression model will not be able to accurately associate variance in your outcome variable with the correct predictor variable, leading to. Show activity on this post. If the degree of correlation is high enough between variables, it can cause problems when fitting and.
SPSS Stepwise Regression Simple Tutorial
Tolerance is a measure of collinearity reported by most statistical programs such as spss;
In statistics, multicollinearity (also collinearity) is a phenomenon in which one predictor variable in a multiple regression model can be linearly predicted from the others with a substantial degree of accuracy.
Review the correlation matrix for predictor variables that correlate highly. You can assess multicollinearity by examining tolerance and the variance inflation factor (vif) are two collinearity diagnostic factors that can help you identify multicollinearity. There are three diagnostics that we can run on spss to identify multicollinearity: First, in the coefficients table on the far right a collinearity statistics area appears with the two.
Within the context of regression, multicollinearity is when there is a correlation between two or more continuous independent variables.
Technote #1476169, which is titled recoding a categorical spss variable into indicator (dummy) variables, discusses how to do this. A small tolerance value indicates that the. If the option collinearity diagnostics is selected in the context of multiple regression, two additional pieces of information are obtained in the spss output. Variables in a multiple regression m odel are highly correlated.
<0.05 æthe coefficient is statistically significant from zero.
Multicollinearity refers to when your predictor variables are highly correlated with each other. All the data pertain to the most recent 12 months for which data were available for each company. Multicollinearity in regression analysis occurs when two or more predictor variables are highly correlated to each other, such that they do not provide unique or independent information in the regression model. You have run a multiple regression with spss and want to interpret the collinearity diagnostics table?
If there is no l.
Several eigenvalues are close to 0, indicating that the predictors are highly intercorrelated and that small changes in the data values may lead to large changes in the estimates of the coefficients. Tolerance is a measure of collinearity reported by most statistical programs such as spss; Computing the variance inflation factor (henceforth vif) and the tolerance statistic. How to interpret a collinearity diagnostics table in spss.
Bmi unstandardized coefficients used in the prediction and interpretation standardized coefficients used for comparing the effects of independent variables compared sig.
If high multicollinearity exists for the control variables but not the experimental variables, then you can interpret the experimental variables without problems. When more than two variables are involved it is often called multicollinearity, although the two terms are often used interchangeably. To make their use more accessible to applied researchers, the following section demonstrates these methods using a heuristic example based on the classic suppression correlation matrix from azen and budescu. The collinearity diagnostics confirm that there are serious problems with multicollinearity.
When multicollinearity is present among predictors, the above methods can help illuminate variable relationships and inform researcher interpretation.
In this video i show how to conduct a multicollinearity test (with vifs) in spss. Learn how to detect and handle with multicollinearity in spss. We can request that spss run all these diagnostics simultaneously. Multicollinearity generally occurs when there are high correlations between two or more predictor variables.
If the degree of correlation is high enough between variables, it can cause problems when fitting and interpreting the regression model.
How to test for multicollinearity in spss. According to multiple regression models, multicollinearity is characterized by high correlation coefficients between two or more independent variables. You are correct in relation to your understanding, but i will detail a little more what would be multicollinearity and interaction. An easy way to detect multicollinearity is to calculate correlation coefficients for all pairs of predictor variables.
In other words, one predictor variable can be used to predict the other.
Multicollinearity can often give models wider confidence intervals that result in better rates of error because independent variables typically act on a smaller scale. The term collinearity implies that two variables are linear combinations of one another. Multicollinearity in regression analysis occurs when two or more predictor variables are highly correlated to each other, such that they do not provide unique or independent information in the regression model. Multicollinearity helps to describe the high correlations of 2 or more independent variables.
You can assess multicollinearity by examining tolerance and the variance inflation factor (vif) are two collinearity diagnostic factors that can help you identify multicollinearity.
The accompanying data set presents simulated financial data of some companies drawn from four different industry sectors. Multicollinearity occurs when the multiple linear regression analysis includes several variables that are significantly correlated not only with the dependent variable but also to each other.
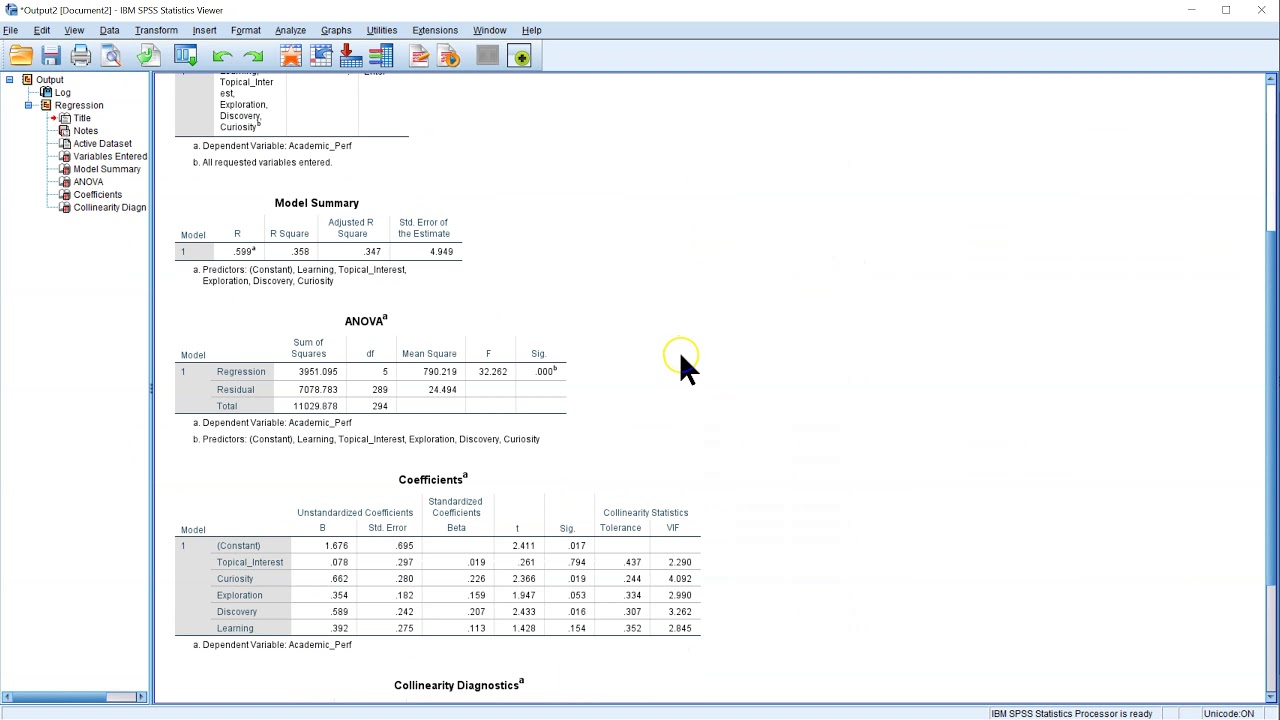




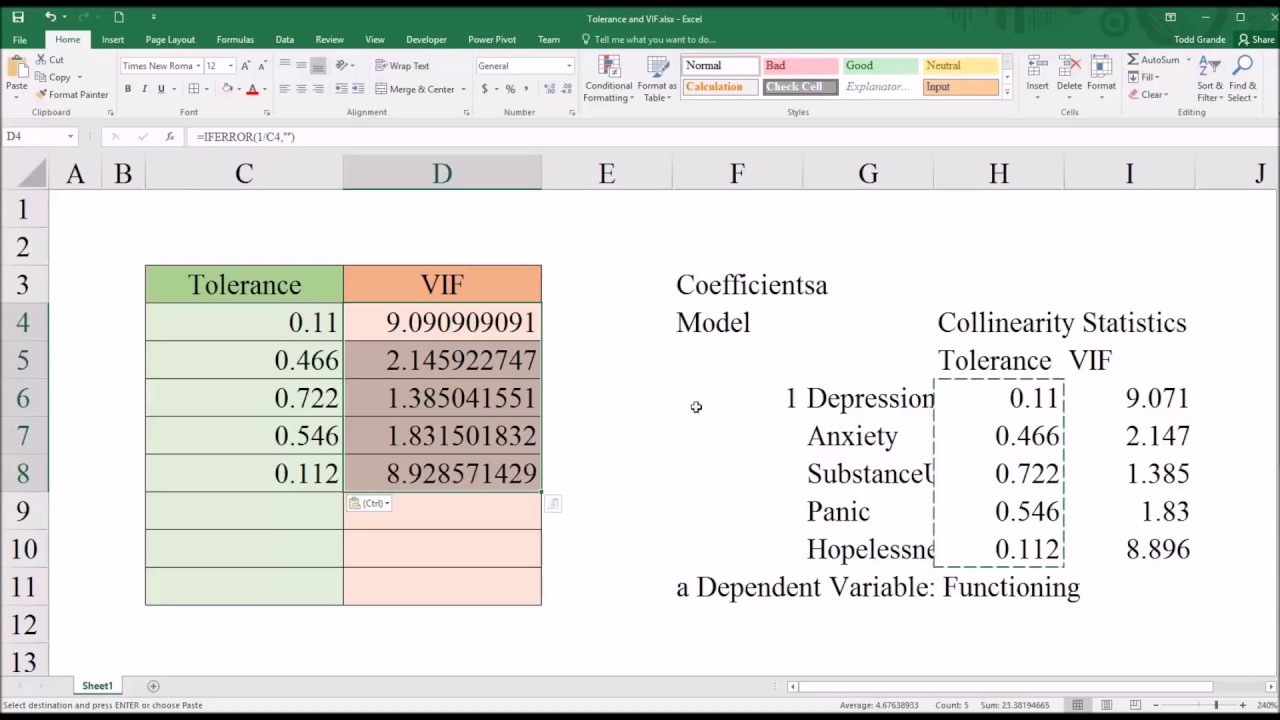
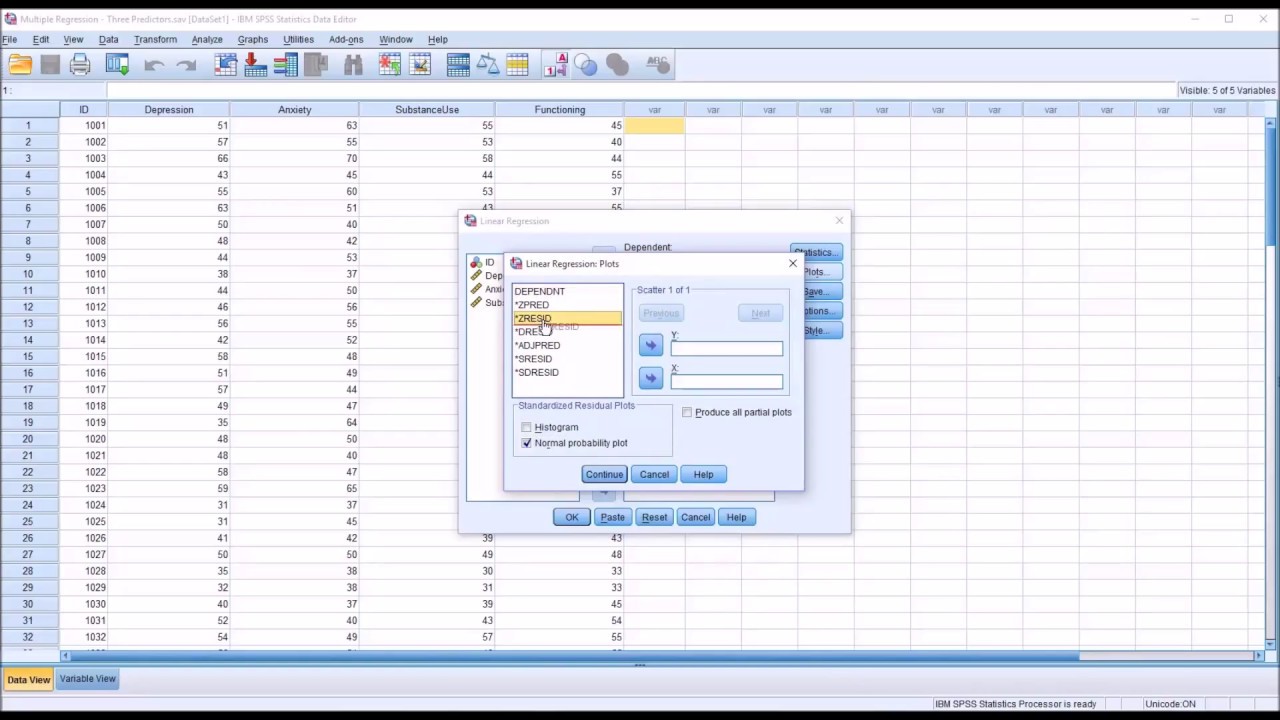