For example, and are perfectly collinear if there exist parameters and such that, for all observations i, we have = +. Multicollinearity refers to a situation in which more than two explanatory variables in a multiple. Multicollinearity used as a noun is very rare.
Multicollinearity Part 1 YouTube
In statistics, multicollinearity occurs when two or more predictor variables are highly correlated with each other, such that they do not provide unique or independent information in the regression model.
A case of multiple regression in which the predictor variables are themselves highly correlated familiarity information:
This usually caused by the researcher or you while creating new predictor variables. Multicollinearity is a statistical phenomenon in which two or more variables in a regression model are dependent upon the other variables in such a way that one can be linearly predicted from the other with a high degree of accuracy. If the degree of this correlation is high, it may cause problems while predicting results from the model. Multicollinearity represents a high degree of linear intercorrelation between explanatory variables in a multiple regression model and leads to incorrect results of regression analyses.
In the process of multiple regression, where the impact of.
Multicollinearity refers to a situation at some stage in which two or greater explanatory variables in the course of a multiple correlation model are pretty linearly related. Multicollinearity is a special case of collinearity where a strong linear relationship exists between 3 or. Correlation refers to the linear relationship between 2 variables. Diagnostic tools of multicollinearity include the variance inflation factor (vif), condition index and condition number, and variance decomposition proportion (vdp).
• multicollinearity (noun) the noun multicollinearity has 1 sense:.
This correlation is not expected as the independent variables are assumed to be independent. In a regression context, multicollinearity can make it difficult to determine the effect of each predictor on the response, and can make it challenging to determine which variables to include in the model. Perfect multicollinearity means that one explanatory variable is an exact linear function of one or more explanatory variables with no error term. Collinearity is a linear association between two explanatory variables.two variables are perfectly collinear if there is an exact linear relationship between them.
Multicollinearity occurs when independent variables in a regression model are correlated.
By jim frost 185 comments. If two or more independent variables have an exact linear relationship between them then we have perfect multicollinearity. When several independent variables are highly but not perfectly correlated among themselves, the regression result is unreliable, this phenomenon is known as multicollinearity, and as a consequence, we are not able to disprove the null hypothesis, wherein we should actually reject the same. Imperfect multicollinearity means that there is a linear relationship between the variables, but there is.
Multicollinearity occurs when two or more independent variables are highly correlated with one another in a regression model.
Multicollinearity is a phenomenon in which one independent variable is highly correlated with one or more of the other independent variables in a multiple regression equation. Including the same information twice (weight in pounds and weight in kilograms), not using dummy variables correctly (falling. The term multicollinearity refers to the condition in which two or more predictors are highly correlated with one another. It describes a perfect or exact relationship between the regression exploratory variables.
This is generally caused due to the experiments designed poorly, methods of collection of data which cannot be manipulated, or purely observational data.
Multicollinearity is a statistical phenomenon in which multiple independent variables show high correlation between each other. This correlation is a problem because independent variables should be independent. This means that an independent variable can be predicted from another independent variable in a regression model. Predictors) have a strong linear relationship.
If the degree of correlation between variables is high enough, it can cause.
Collinearity refers to a problem when running a regression model where 2 or more independent variables (a.k.a. It describes a perfect or exact relationship between the regression exploratory variables. The existence of such a high degree of correlation between supposedly independent variables being used to estimate a dependent variable that the contribution of each independent variable to variation in. In a few cases, the.
Why is multicollinearity a problem?
It generally occurs when the independent variables in a regression model are correlated with each other. In other words, one independent variable can be linearly predicted from one or multiple other independent variables with a substantial degree of certainty.
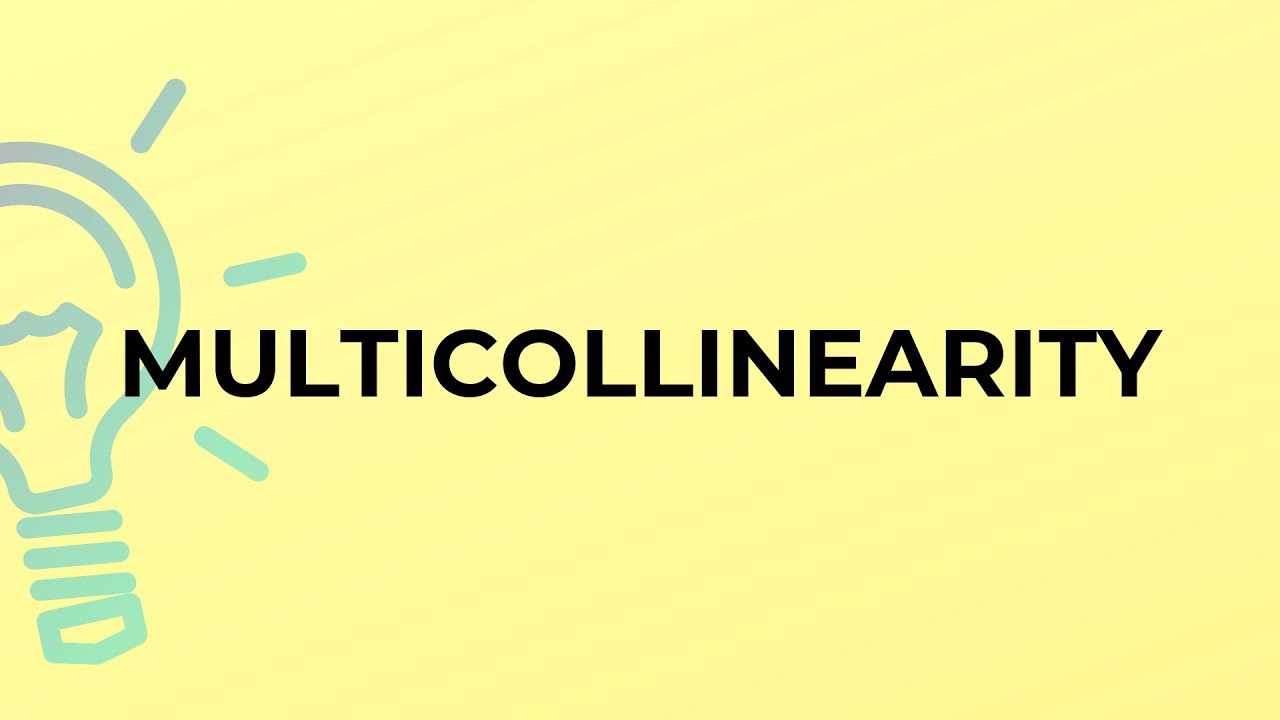
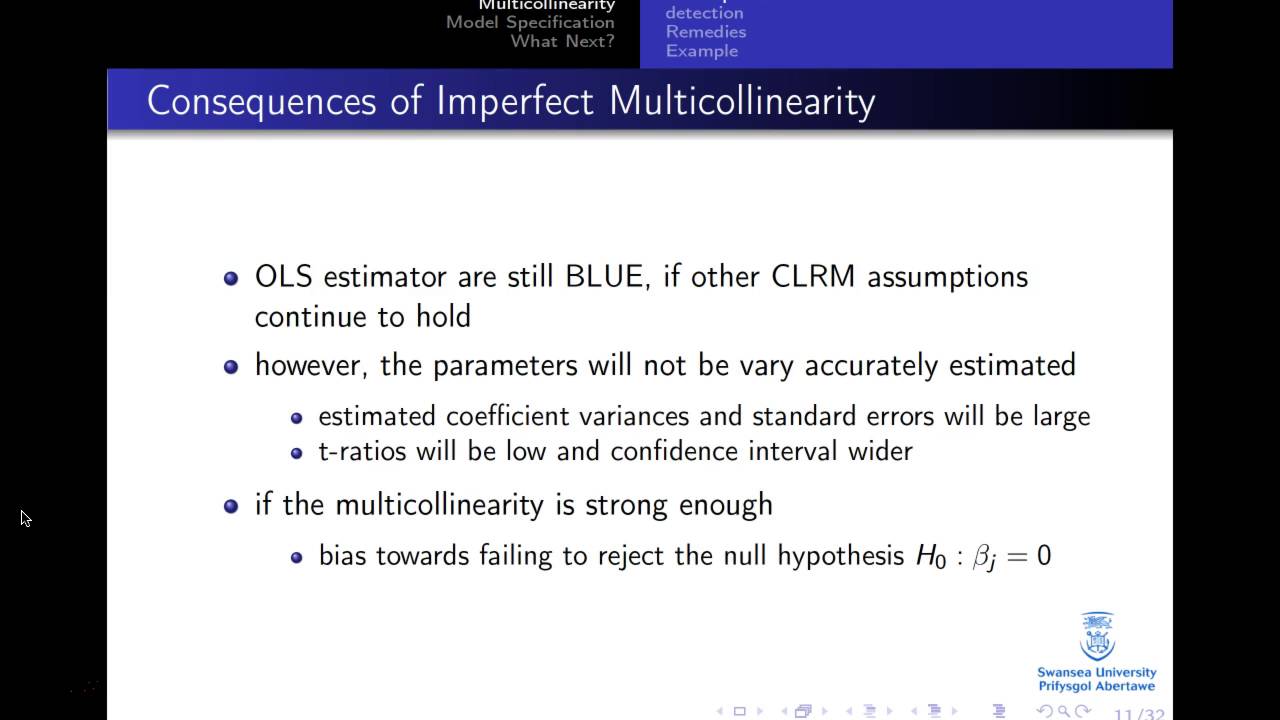

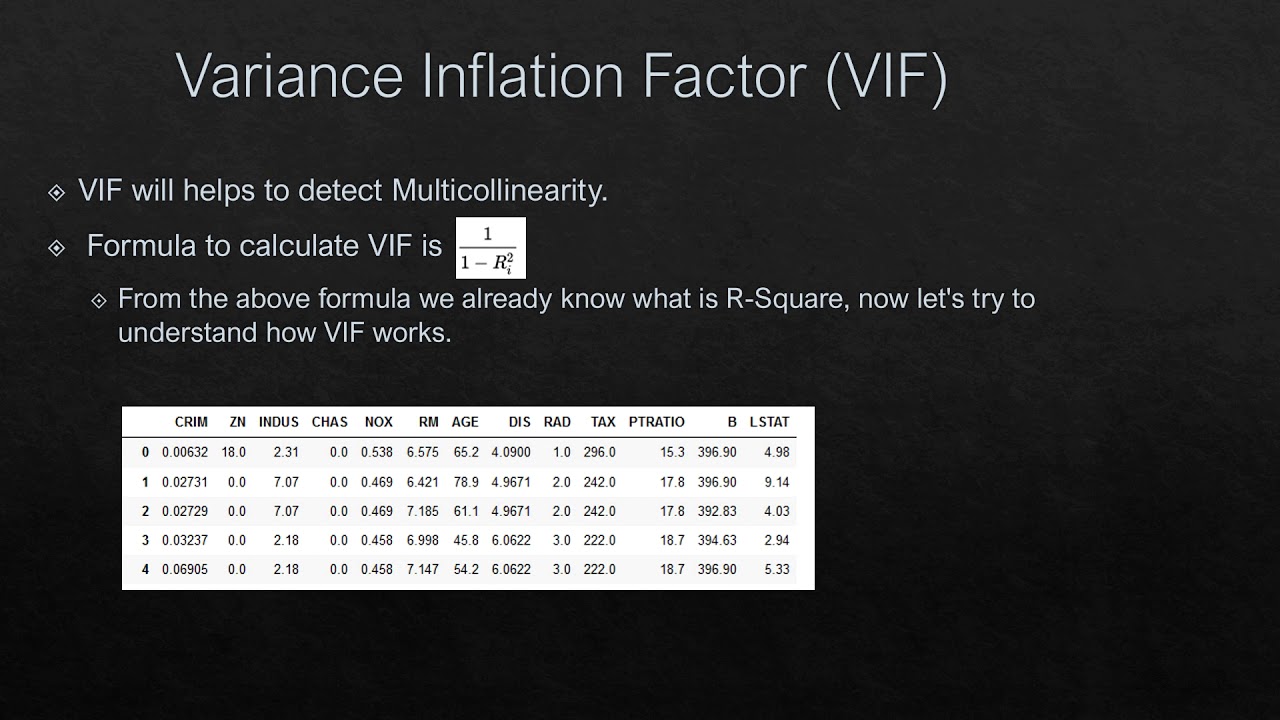


