If two or more independent variables are too correlated, the data obtained from the regression will be disturbed because the independent variables are actually dependent between each other. If the degree of correlation is high enough between variables, it can cause problems when fitting and. If the degree of correlation between variables is high enough, it can cause.
Why Multicollinearity is a problem and How to Detect it in
For example, and are perfectly collinear if there exist parameters and such that, for all observations i, we have = +.
Every regression analysis has a goal to find and isolate the relationship between each independent variable and.
Collinearity is an undesired situation for any statistical regression model since it diminishes the reliability of the model itself. If the degree of correlation is high enough between variables, it can cause problems when fitting and interpreting the regression model. Imperfect multicollinearity means that there is a linear relationship between the variables, but there is. If there is no linear relationship between predictor variables, they are said to be orthogonal [2].
Multicollinearity occurs when independent variables in a regression model are correlated.
This correlation is a problem because independent variables should be independent. Collinearity refers to a problem when running a regression model where 2 or more independent variables (a.k.a. Multicollinearity occurs when the multiple linear regression analysis includes several variables that are significantly correlated not only with the dependent variable but also to each other. Content from this work may be used under the terms of the creative commons.
Multicollinearity in regression is a condition that occurs when some predictor variables in the model are correlated with other predictor variables.
Perfect multicollinearity means that one explanatory variable is an exact linear function of one or more explanatory variables with no error term. Multicollinearity is a statistical phenomenon in which two or more variables in a regression model are dependent upon the other variables in such a way that one can be linearly predicted from the other with a high degree of accuracy. What is the definition of multicollinearity? Multicollinearity is the occurrence of high intercorrelations among two or more independent variables in a multiple regression model.
A guide to multicollinearity & vif in regression.
Multicollinearity can lead to skewed or misleading results. Other things being equal, the larger the standard error of a regression coefficient, the less likely it is that this coefficient will be statistically significant. In statistics, multicollinearity occurs when two or more predictor variables are highly correlated with each other, such that they do not provide unique or independent information in the regression model. Collinearity is a linear association between two explanatory variables.two variables are perfectly collinear if there is an exact linear relationship between them.
If the degree of this correlation is high, it may cause problems while predicting results from the model.
This correlation is not expected as the independent variables are assumed to be independent. In statistics, multicollinearity (also collinearity) is a phenomenon in which one predictor variable in a multiple regression model can be linearly predicted. Exact collinearity is a perfect linear relationship between two explanatory variables x 1 and x 2.in other words, exact collinearity occurs if one variable determines the other variable (e.g., x 1 = 100 − 2x 2).if such relationship exists between more than two explanatory variables (e.g., x 1 = 100 − 2x 2 + 3x 3), the relationship is defined as. A phenomenon in which two or more predictor variables in a multiple regression model are highly correlated, so that the coefficient estimates may change erratically in response to small changes in the model or data.
Predictors) have a strong linear relationship.
Multicollinearity is a special case of collinearity where a strong linear relationship exists between 3 or more independent variables even if no pair of variables has a high correlation. It is generally used in observational studies and less popular in experimental studies. Variables in a multiple regression m odel are highly correlated. Why is multicollinearity a potential problem?
It generally occurs when the independent variables in a regression model are correlated with each other.
Multicollinearity is a problem because it undermines the statistical significance of an independent variable. If there is no l. Severe multicollinearity is problematic because it can increase the variance of the regression coefficients, making them unstable. Multicollinearity in regression analysis occurs when two or more predictor variables are highly correlated to each other, such that they do not provide unique or independent information in the regression model.
When several independent variables are highly but not perfectly correlated among themselves, the regression result is unreliable, this phenomenon is known as multicollinearity, and as a consequence, we are not able to disprove the null hypothesis, wherein we should actually reject the same.
By jim frost 185 comments.




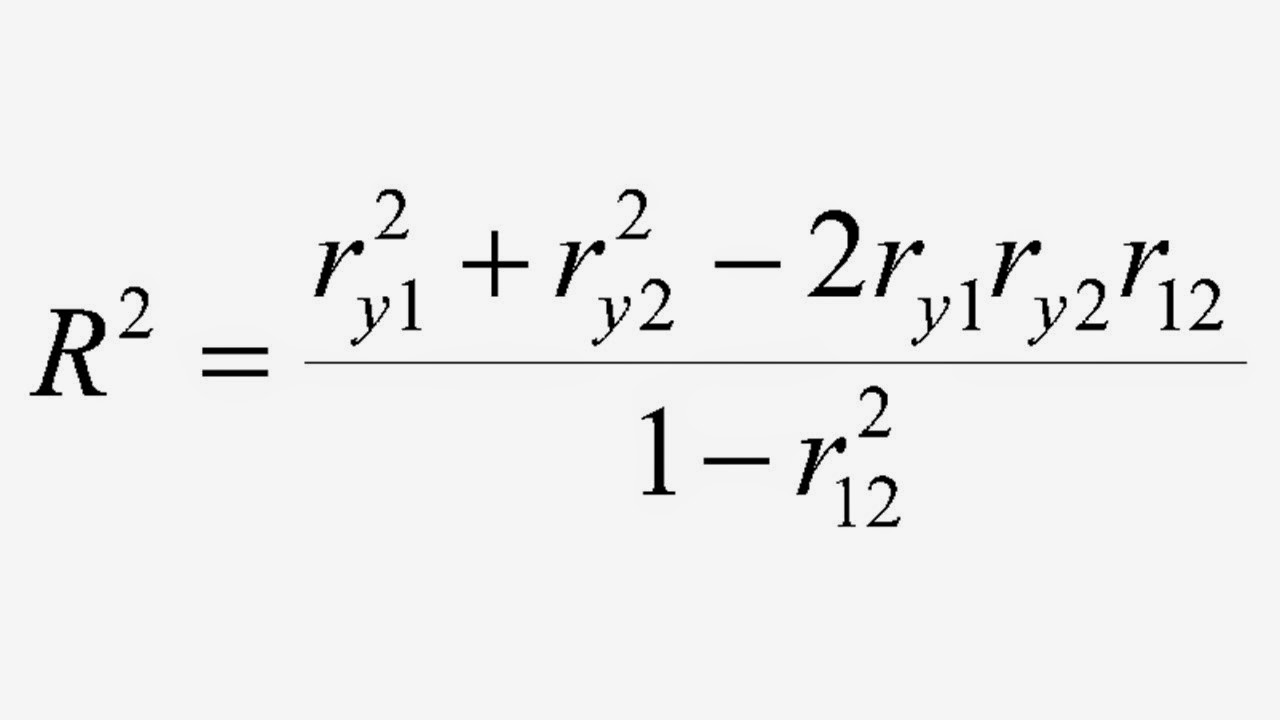

