For each of these predictor examples, the researcher just observes the values as they occur for the people in the random sample. Instead, they are on very similar or relating inputs. Multicollinearity happens more often than not in such observational studies.
PPT MULTICOLLINEARITY PowerPoint Presentation, free
For investing, it is a common consideration when performing technical analysis to imagine probable future price movements of a security, like a stock or a commodity future.
For example, an individual's blood pressure is not collinear with age, but also weight, stress, and.
5 ü e ú 6 : However, producing a regression output using excel gives num error because this dataset contains a violation of. The examples for multicollinear predictors would be the sales price and age of a car, the weight, height of a person, or annual income and years of education. Multicollinearity test example using spss| after the normality of the data in the regression model are met, the next step to determine whether there is similarity between the independent variables in a model it is necessary to multicollinearity test.
For example, if you square term x to model curvature, clearly there is a correlation between x and x 2.
Examples of multicollinearity survival analysis multicollinearity may represent a serious issue in. Let’s take an example of loan data. This creates redundant information, skewing the results in a regression model. Ü l ú 4 e ú 5 :
In other words, it’s a byproduct of the model that we specify rather than being present in the data itself.
You can also locate that multicollinearity may be a characteristic of the making plans of the test. A special procedure is recommended to assess the impact of multicollinearity on the results. One such context is human biology. Below are the examples to implement multicollinearity:
Obvious examples include a person's gender, race, grade point average, math sat score, iq, and starting salary.
This type of multicollinearity is present in the data itself rather than being an artifact of our model. This data set includes measurements of 252 men. If you detect multicollinearity, the next step is to decide if you need to resolve it in some way. Examples of multicollinearity example #1 let’s assume that abc ltd, a kpo, has been hired by a pharmaceutical company to provide research services and.
Market analysts want to avoid using the technical indicators that are collinear.
In the material manufacturer case, we can without problems see that advertising and marketing and volume are correlated predictor variables, main to foremost swings inside the impact of marketing while the quantity is and aren’t included within the version. Here is an example of perfect multicollinearity in a model with two explanatory variables: Meaning nature of multicollinearity perfectmulticollinearityi y i = 1 + 2x 2i + 3x 3i + + kx ki +u i (1) if,forexample,x 2i +3x 3i = 1wehaveperfectcollinearityfor x 2i = 1 3x 3i. For illustration, we take a look at a new example, bodyfat.
Including the same information twice (weight in pounds and weight in kilograms), not using dummy variables correctly (falling into the dummy variable trap), etc.
Multicollinearity happens more often than not in such observational studies. Calculating correlation coefficients is the easiest way to detect multicollinearity for. We can find out the value of x1 by (x2 + x3). Similarities between the independent variables will result in a very strong correlation.
Examples of correlated predictor variables (also called multicollinear predictors) are:
Obvious examples include a person's gender, race, grade point average, math sat score, iq, and starting salary. For this example, the output shows multicollinearity with volume and ads, but not with price and location. In sas, when we run proc regression we add ‘/vif tol’ in the code. You may find that the multicollinearity is a function of the design of the experiment.
The goal of the study was to develop a model, based on physical measurements, to predict percent body fat.
Multicollinearity is also observed in many other contexts. For example, in the cloth manufacturer case, we saw that advertising and volume were correlated predictor variables, resulting in major swings in the impact of advertising when volume was and was not included in the model. This indicates that there is strong multicollinearity among x1, x2 and x3. In other words, one predictor variable can be used to predict the other.
Our independent variable (x1) is not exactly independent.
Here, as we can see x2 = 3 x x1 and x3 = 2 x x1, therefore, all the independent variables correlated as per the. If x1 = total loan amount, x2 = principal amount, x3 = interest amount. Where the hats on the variances and covariances indicate that they are sample, not population, quantities. This is a classic example of multicollinearity causing the coefficient estimates to appear a bit whacky and unintuitive.
Interest rates for different terms to maturity in various situations it might be.
For each of these predictor examples, the researcher just observes the values as they occur for the people in her random sample.
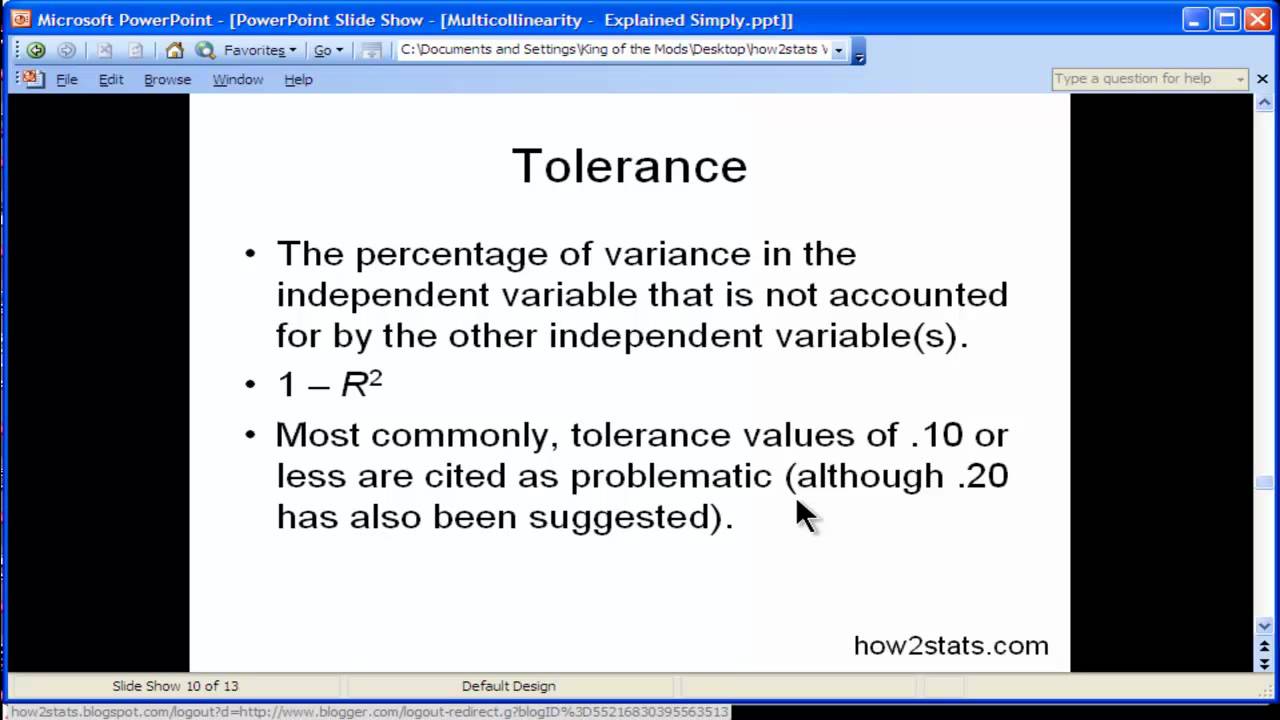




