Here, as we can see x2 = 3 x x1 and x3 = 2 x x1, therefore, all the independent variables correlated as per the definition of multicollinearity, 6 ü e ý ü : Last updated over 2 years ago;
Learn to Test for Multicollinearity in SPSS With Data From
This example demonstrates how to test for multicollinearity specifically in multiple linear regression.
In this case, the variable “single” is a perfect linear combination of the “married” and “divorced” variables.
Multicollinearity essentials and vif in r. Let’s assume that abc ltd, a kpo, has been hired by a pharmaceutical company to provide research services and statistical analysis on the diseases in india. I'am trying to do a multinomial logistic regression with categorical dependent variable using r, so before starting the logistic regression i want to check multicollinearity with all independents variables expressed as dichotomous and ordinal. The standardised determinant for three variables case is written as.
This shows that 58% of the variation in volume can be explained by the other variables.
You may find that the multicollinearity is a function of the design of the experiment. It means that independent variables are linearly correlated to each other and they are numerical in nature. Our independent variable (x1) is not exactly independent. Multicollinearity refers to a situation in which two or more explanatory variables in a multiple regression model are highly linearly related.”
Here is an example of perfect multicollinearity in a model with two explanatory variables:
For example, if you square term x to model curvature, clearly there is a correlation between x and x 2. The hypothesis to be tested at this step is as under: Ü l ú 4 e ú 5 : Provides further evidence of the above claims.
Multicollinearity in regression analysis occurs when two or more predictor variables are highly correlated to each other, such that they do not provide unique or independent information in the regression model.
Rxixj.x1x2….xk ≠ 0 h 0: If x1 = total loan amount, x2 = principal amount, x3 = interest amount. In other words, it’s a byproduct of the model that we specify rather than being present in the data itself. Multicollinearity example n = 25 males;
For this, abc ltd has selected age, weight, profession, height, and health as the prima facie parameters.
And, weight and bsa appear to be strongly related (r = 0.875), while stress and bsa appear to be hardly related at all (r = 0.018). For the statistical model involving more than three explanatory variables, we can develop similar formula for partial correlation coefficients. This type of multicollinearity is present in the data itself rather than being an artifact of our model. X k = 0 h 0:
R x i x j.
For example, in the cloth manufacturer case, we saw that advertising and volume were correlated predictor variables, resulting in major swings in the impact of advertising when volume was and was not included in the model. | 1 r x 1 x 2 r x 1 x 3 r x 1 x 2 1 r x 2 x 3 r x 1 x 3 r x 2 x 3 1 |. The categorical variables are either ordinal or nominal in nature hence we cannot say that they can be linearly correlated. 5 ü e ú 6 :
Blood pressure appears to be related fairly strongly to weight (r = 0.950) and bsa (r = 0.866), and hardly related at all to stress level (r = 0.164).
Variance inflation factor (vif) is used for detecting the multicollinearity in a model, which measures the correlation and strength of correlation between the independent variables in a regression model. Below are the examples to implement multicollinearity: Height leftfoot rtfoot height 1.0000000 0.5466786 0.5345347 leftfoot 0.5466786 1.0000000 0.9078141 rtfoot 0.5345347 0.9078141 1.0000000 note the strong correlation between the feet left foot only: We can find out the value of x1 by (x2 + x3).
Including the same information twice (weight in pounds and weight in kilograms), not using dummy variables correctly (falling into the dummy variable trap), etc.
If the degree of correlation is high enough between variables, it can cause problems when fitting and interpreting the regression model. The high correlation among some of the predictors suggests. You can also locate that multicollinearity may be a characteristic of the making plans of the test. Let’s take an example of loan data.
The high correlation among some of the predictors suggests.
If we attempt to fit a multiple linear regression model in r using this dataset, we won’t be able to produce a coefficient estimate for every predictor variable: Provides further evidence of the above claims. 2 an example in r: This situation is referred as collinearity.
Now in case of perfect multicollinearity the simple correlation coefficients are equal to unity and so the above determinant turns to zero.
X2i = λ0 + λ1 * x1i. In the above example, there is a multicollinearity situation since. That is, | 1 1 1 1 1 1 1 1 1 | = 0. Blood pressure appears to be related fairly strongly to weight (r = 0.950) and bsa (r = 0.866), and hardly related at all to stress level (r = 0.164).
For example, x1 and x2 are perfectly collinear if there exist parameters λ0 and λ1 such that, for all observations i, we have.
There is an extreme situation, called multicollinearity, where collinearity exists between three or more variables even if no pair. Height is in inches, rtfoot and leftfoot are foot lengths in centimeters correlation matrix: R x i x j. Testing for multicollinearity when there are factors (1 answer) closed 5 years ago.
The multicollinearity is the term is related to numerical variables.
This indicates that there is strong multicollinearity among x1, x2 and x3. This is an example of perfect multicollinearity. In the material manufacturer case, we can without problems see that advertising and marketing and volume are correlated predictor variables, main to foremost swings inside the impact of marketing while the quantity is and aren’t.



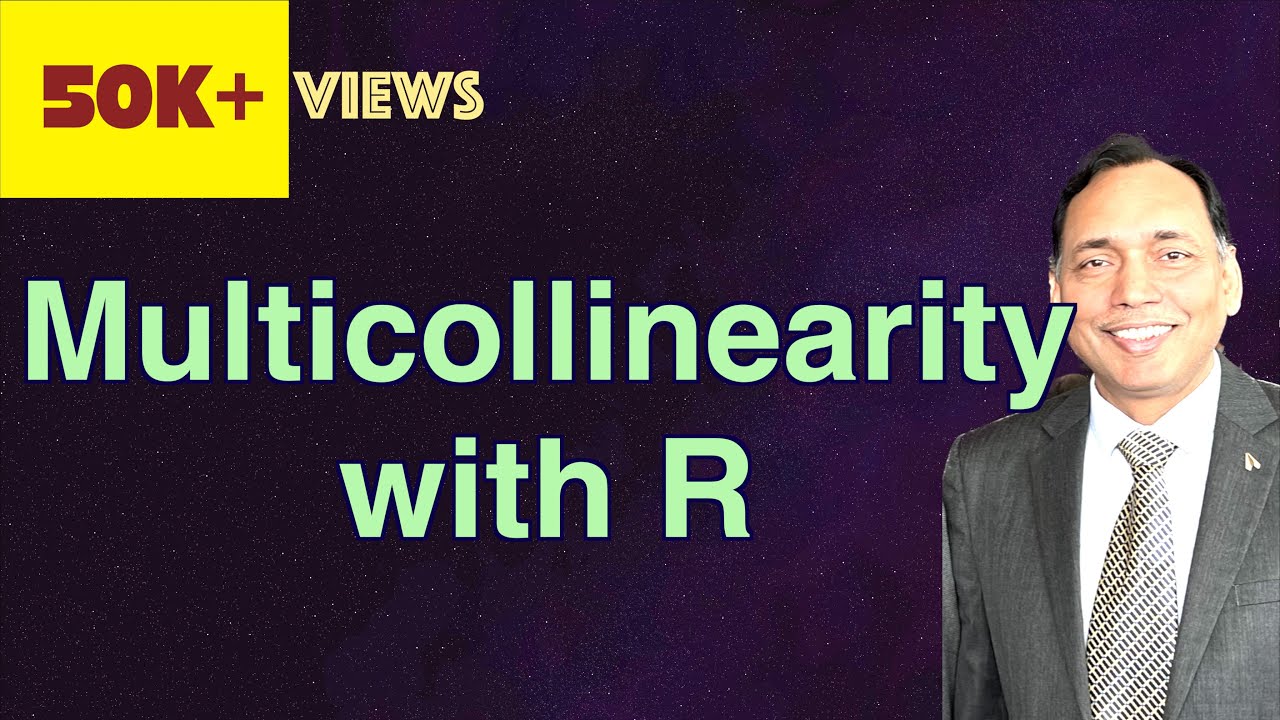


