That's why many regression analysts often rely on what are called variance inflation factors ( vif) to help detect multicollinearity. How are shadow prices used in the economy? It is possible that the pairwise correlations are small, and yet a linear dependence exists among three or even more variables, for example, \ (x_3 = 2x_1 + 5x_2 + \text {error}\), say.
Data Visualization What is the best way to present
Both the example1 and the example2 sheets demonstrate perfect multicollinearity.
The focus of the course is on understanding and application, rather than detailed mathematical derivations.
Wildly different coefficients in the two models could be a sign of multicollinearity. The following examples show the three most common scenarios of perfect multicollinearity in practice. Multicollinearity is a term used in data analytics that describes the occurrence of two exploratory variables in a linear regression model that is found to be correlated through adequate analysis and a predetermined degree of accuracy. S&p 500® index replicating etf (ticker symbol:
Change b1 and then use solver to find a least squares solution.
Look for instability of the coefficients. Treasury note yield, merrill lynch u.s. In the material manufacturer case, we can without problems see that advertising and marketing and volume are correlated predictor variables, main to foremost swings inside the impact of marketing while the quantity is and aren’t. We can find out the value of x1 by (x2 + x3).
How to find multicollinearity in scatterplot example?
Review scatterplot and correlation matrices. This video explains how to use microsoft excel to calculate the correlation coefficients and interprets the results. This is an extension of the simple case of collinearity between two variables. All these are introduced and explained using easy to understand examples in microsoft excel.
This is an extension of the simple case of collinearity between two variables.
Variables are said to be multicollinear if there is a linear relationship between them. For example, for three variables x1, x2 and x3, we say that they are multicollinear if we can write: Treasury bill yield, 10 years u.s. In example2, there is a slightly more complicated linear relationship between the two x variables.
Coefficients on different samples are wildly different.
There is something called variance inflation factor (vif), if vif value is greater than ten (vif > 10) then there is a collinearity problem. The data for the first 12 states in example 1 of multiple correlation ). For this example, the output shows multicollinearity with volume and ads, but not with price and location. Spy) adjusted close prices arithmetic monthly returns, 1 year u.s.
Vif(r1, j) = vif of the jth variable for the data in range r1.
Suppose we want to use “height in centimeters” and “height in meters” to predict the weight of a certain species of dolphin. This course uses the ‘data analysis’ tool box which is standard with the windows version of microsoft excel. Also, find the prediction model equation in this video tutorial and explanation of model and assumptions. Look for incorrect coefficient signs.
In sas, when we run proc regression we add ‘/vif tol’ in the code.
In example1, one variable is directly proportional to a second variable. High yield corporate bond index yield effective monthly yields, u.s. One predictor variable is a multiple of another. Review the variance inflation factor.
Fortunately, it’s possible to detect multicollinearity using a metric known as the variance inflation factor (vif), which measures the correlation and strength of correlation between the explanatory variables in a regression model.
That said, it could be multicollinearity and warrants taking a second look at other indicators. This indicates that there is strong multicollinearity among x1, x2 and x3. This tutorial explains how to calculate vif in excel. Check the tolerance and vif for the data displayed in figure 1 of multiple correlation (i.e.
If you have a large enough sample, split the sample in half and run the model separately on each half.
This type of multicollinearity is present in the data itself rather than being an artifact of our model. Here’s what our dataset might look like: You may find that the multicollinearity is a function of the design of the experiment. Where a and b are real numbers.
Examples of multicollinearity example #1 let’s assume that abc ltd, a kpo, has been hired by a pharmaceutical company to provide research services and.
If x1 = total loan amount, x2 = principal amount, x3 = interest amount. X1 = ax2 + bx3. You can also locate that multicollinearity may be a characteristic of the making plans of the test. Multicollinearity happens more often than not in such observational studies.
For example, in the cloth manufacturer case, we saw that advertising and volume were correlated predictor variables, resulting in major swings in the impact of advertising when volume was and was not included in the model.
Obvious examples include a person's gender, race, grade point average, math sat score, iq, and starting salary. The variables are independent and are found to be correlated in some regard. Let’s take an example of loan data. Variables are said to be multicollinear if there is a linear relationship between them.
This video explains how to assist the problem in a formal way.
Where a and b are real numbers. Our independent variable (x1) is not exactly independent. For each of these predictor examples, the researcher just observes the values as they occur for the people in the random sample. X1 = ax2 + bx3.
For example, if you square term x to model curvature, clearly there is a correlation between x and x 2.
For example, for three variables x1, x2 and x3, we say that they are multicollinear if we can write:


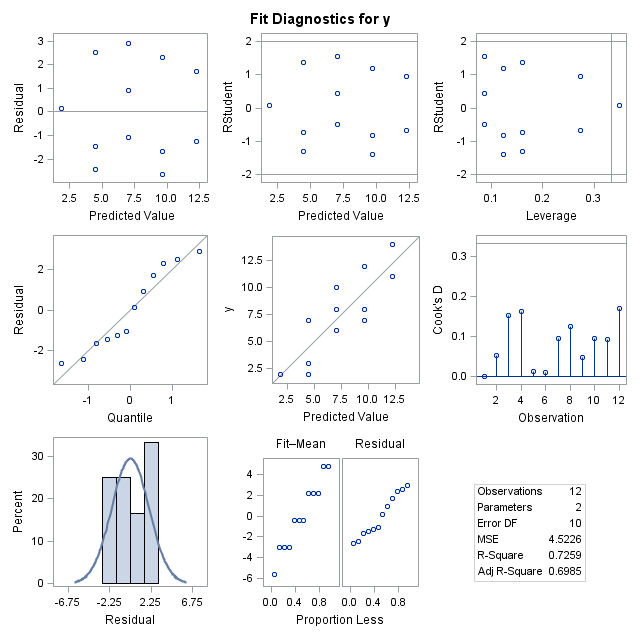


